Effect of Long-Term Agricultural Management on the Soil Microbiota Influenced by the Time of Soil Sampling
- 1Instituto de Investigaciones en Biodiversidad y Biotecnología (INBIOTEC), Consejo Nacional de Investigaciones Científicas y Técnicas (CONICET), Mar del Plata, Buenos Aires, Argentina
- 2Julius Kühn Institute (JKI) – Federal Research Centre for Cultivated Plants, Institute for Epidemiology and Pathogen Diagnostics, Braunschweig, Germany
- 3Leibniz Institute of Vegetable and Ornamental Crops (IGZ), Plant-Microbe Systems, Großbeeren, Germany
- 4Department of Agriculture, Ecotrophology and Landscape Development, Institute of Bioanalytical Sciences (IBAS), Anhalt University of Applied Sciences, Bernburg, Germany
- 5Department of Nutritional Crop Physiology, Institute of Crop Science, University of Hohenheim, Stuttgart, Germany
- 6Section of Microbiology, Department of Biology, University of Copenhagen, Copenhagen, Denmark
Application of agrochemicals and mechanization enabled increasing agricultural productivity yet caused various environmental and soil health-related problems. Agricultural practices affect soil microorganisms, which are the key players of many ecosystem processes. However, less is known about whether this effect differs between time points. Therefore, soil was sampled in winter (without crop) and in summer (in the presence of maize) from a long-term field experiment (LTE) in Bernburg (Germany) managed either under cultivator tillage (CT) or moldboard plow (MP) in combination with either intensive nitrogen (N)-fertilization and pesticides (Int) or extensive reduced N-fertilization without fungicides (Ext), respectively. High-throughput sequencing of 16S rRNA gene and fungal ITS2 amplicons showed that changes in the microbial community composition were correlated to differences in soil chemical properties caused by tillage practice. Microbial communities of soils sampled in winter differed only depending on the tillage practice while, in summer, also a strong effect of the fertilization intensity was observed. A small proportion of microbial taxa was shared between soils from the two sampling times, suggesting the existence of a stable core microbiota at the LTE. In general, taxa associated with organic matter decomposition (such as Actinobacteria, Bacteroidetes, Rhizopus, and Exophiala) had a higher relative abundance under CT. Among the taxa with significant changes in relative abundances due to different long-term agricultural practices were putative pathogenic (e.g., Gibellulopsis and Gibberella) and beneficial microbial genera (e.g., Chitinophagaceae, Ferruginibacter, and Minimedusa). In summary, this study suggests that the effects of long-term agricultural management practices on the soil microbiota are influenced by the soil sampling time, and this needs to be kept in mind in future studies for the interpretation of field data.
Introduction
Agricultural production has been intensified globally through the use of irrigation, fertilizers, biocides, and mechanization to meet the growing demands for food, feed, and fiber (1). However, intensive agricultural management contributes to soil erosion, salinization, nutrient depletion, and imbalance and a decline in water-holding capacity and soil structure (2). Furthermore, loss of soil biodiversity as well as increases of soil-borne plant pathogens are reported as principal consequences of conventional intensive agriculture (3, 4). Applying a sustainable crop management, e.g., highly diverse crop rotations and reduced tillage coupled with lower agrochemical input, is suggested to conserve soil quality and health as well as biodiversity (5, 6).
Soil properties in agricultural systems are affected by multiple environmental and anthropogenic factors, resulting in temporal variability, i.e., variability between years and within growing seasons (7, 8). In particular, biological soil properties are highly spatiotemporally variable. For instance, temporal dynamics of soil microbial communities and fluctuations in microbial (relative) abundances during the growing season of a crop have been reported (9–12). In contrast, other features, such as soil pH, texture, and porosity, are considered to be rather static (8).
Soil microorganisms are involved in nutrient cycling, organic matter (OM) decomposition, pathogen suppression, and maintenance of the soil structure (13). Therefore, the soil microbiota is essential for soil ecosystem functioning and for plant growth and health (14). There is increasing evidence that agricultural management practices affect the soil microbial community structure and composition (12, 15–18). Previous studies show that the type of tillage is one of the main drivers of the microbial community composition (19–21), leading to changes in the relative abundance of certain taxa. For instance, actinobacterial taxa (e.g., Nocardioides, Rubrobacter), known to contribute to OM decomposition (22), exhibited a higher relative abundance in soils under cultivator tillage (CT), whereas acidobacterial taxa (such as order Gp4) were higher under conventional moldboard plow (MP) tillage (21). Regarding putative plant pathogens, tillage practices exert different effects on soil microbial communities. For instance, Fusarium or Phoma were shown to be enhanced under CT or MP practices, respectively (20). Fertilizer quantity and quality as well as pesticide input also shape the soil microbiota (20, 21, 23, 24). Nitrogen (N) fertilization intensity was shown to increase the relative abundance and community structure of bacteria and fungi (25) as well as of plant beneficial microorganisms, such as arbuscular mycorrhizal fungi (AMF) (20). Many studies usually focus on the soil microbiota of one growing season disregarding temporal variability between years. This is of particular concern as an improved understanding of the temporal dependency of the farming practice effect on the soil microbiota under field conditions could also help to understand the mechanism behind plant–soil feedback and agricultural legacies (26, 27). This could make a critical contribution to the development of microbial-based solutions for sustainable farming practices.
In the present study, we used a long-term field experiment (LTE) established in Bernburg (Saxony-Anhalt, Germany) in 1992. This LTE facilitates comparing two different tillage practices, i.e., CT vs. MP. Additionally, two different intensities of N-fertilization and pesticide use, i.e., standard N-fertilization with pesticide application (Int) vs. reduced N-fertilization without growth regulators/fungicides (Ext), are applied per tillage practice. The effects of these agricultural managements on the LTE soil microbiota have already been well described (18, 20, 21), but previous studies lack information on the temporal variability of the soil microbiota. Therefore, the objectives of the present study are a) to determine the effect of tillage and N-fertilization intensity on the soil microbiota (here: bacteria, archaea, and fungi) depending on the soil sampling time and b) to evaluate whether soils under different management and sampling time share common microorganisms (core microbiota). We hypothesized that (i) tillage practice is the main driver of the soil microbiota in winter while, in summer, also the fertilization intensity exerts a strong effect. Moreover, we hypothesized that (ii) long-term conservation practices (CT, Ext) exhibit a higher microbial diversity and more potentially beneficial microbes compared with the conventional practices (MP, Int) independent of the sampling time.
Materials and Methods
Site Description and Soil Sampling
The LTE is located at the Anhalt University of Applied Sciences, Bernburg, Saxony-Anhalt, Germany, and it was established in 1992 to evaluate an annual rotation system consisting of winter wheat (Triticum aestivum L.) / maize (Zea mays L.) / winter wheat / winter barley (Hordeum vulgare L.) / winter rapeseed (Brassica napus L.) under two different tillage practices and fertilization intensities. The LTE consists of five plots (1.2 ha each, divided into four subplots [replicates]). The experimental station (51.82°N and 11.70°E, 511 mm mean annual rainfall, 9.7°C mean temperature [1981–2010], 80 m above sea level) was previously described (28). Briefly, the soil is a loess chernozem over limestone (22% clay, 70% silt, and 8% sand) in the plowed upper horizon (20).
Two different tillage practices [CT (12–15 cm depth) vs. MP (20–30 cm depth)] are applied in combination with two intensities of N-fertilization (Int vs. Ext). This results in four treatments (CT.Ext, CT.Int, MP.Ext, and MP.Int), each with four replicates (Table 1).
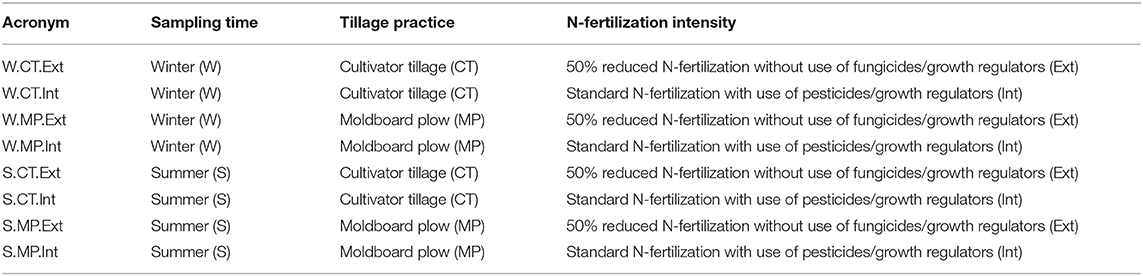
Table 1. Overview of long-term agricultural practices (treatments; n = 4 replicates) studied at LTE Bernburg.
Soil sampling was carried out in the season 2018/2019 in the field used for maize cultivation. The preceding crop was winter wheat (after rapeseed), which was harvested in July 2018. Soil management with CT or MP was applied on 5 November and soil samples were collected on 28 November 2018, from the fallow field (in the following referred to as winter sampling). Maize (cv. Benedictio) was sown on 23 April 2019, with a single-grain seed drill. After sowing, 100 or 40 N kg ha−1 in a water solution of urea and ammonium nitrate (UAN 28; 14% N as carbamide, 7% N as , 7% N as ) were applied as Int or Ext treatment, respectively. As postemergence herbicides, S-metolachlor, atrazine with mesotrione, and prosulfuron were applied two months before soil sampling in both Int and Ext treatments. The second soil sampling was carried out on 2 July 2019, at the vegetative stage of maize [stem elongation 31–34 BBCH scale (29)], in the following referred to as summer sampling.
For each sampling and treatment, 20 random subsamples per replicate (n = 4) were taken with a soil corer (5 cm diameter) from 0 to 20 cm depth in winter. In order to reduce the effect of the standing crop in summer, the soil loosely adhering to maize roots was sampled which was obtained by digging out and shaking nine plants per replicate. Soil subsamples were combined per replicate and homogenized by sieving (mesh size 2 mm). Thus, a total number of 16 samples (four treatments, each four replicates) for each sampling time were collected and stored at −20°C until total community-DNA (TC-DNA) extraction.
Soil Chemical Properties
Total N (TN), total C (TC), OM (for soils sampled in summer), total organic C (TOC), K2O, P2O5, and pH were analyzed according to standard protocols of VDLUFA and DIN (Association of German Agricultural Analytic and Research Institutes e. V. and German Institute for Standardization, respectively). Soil OM was converted to TOC using a conversion factor of 1.724 (30). K2O and P2O5 were converted into K and P, respectively, according to their molecular mass.
Total Community-DNA Extraction
The TC-DNA was extracted from 0.5 g of soil (fresh weight) from each replicate by harsh lysis using a FastPrep-24 bead-beating system and the FastDNA Spin Kit for Soil and then purified using GeneClean Spin Kit according to the manufacturer's instructions (both MP Biomedicals, Santa Ana, California, USA). The TC-DNA quality and yields were checked by 1% agarose gel electrophoresis using 0.5X TBE buffer and stained with 0.005% ethidium bromide. The extracted and purified TC-DNA was stored at −20°C.
Quantification of Bacterial 16S rRNA Gene and Fungal ITS Fragment Copies by Quantitative Real-Time PCR (qPCR)
Quantification of the bacterial 16S rRNA gene was carried out using the primer pair Bact1369F (5'-CGGTGAATACGTTCYCGG-3') and Prok1492R (5'-GGWTACCTTGTTACGACTT-3') (31). The detection of bacterial genes was based on the release of a fluorescence signal from the TaqMan-probe TM1389F [5'-CTTGTACACACCGCCCGTC-3'; (31)] containing the FAM fluorophore attached to the 5'-end and a TAMRA quencher at the 3'-end. Amplifications were performed in 50 μL reaction volume as described in Vogel et al. (32) with the modification of using 1.25 U Hot Start Taq Polymerase (New England BioLabs, Inc., Ipswich, Massachusetts, USA). Serial dilutions of the gel-purified 16S rRNA gene from Escherichia coli (1,467 bp) cloned into pGEM-T vector (Promega, Fitchburg, Wisconsin, USA; optical density OD260 = 0.513) were used for the generation of standard curves (average efficiency = 112.3%; R2 = 0.982).
Fungal ITS fragments were quantified according to the protocol established by Gschwendtner et al. (33) with the primers ITS1 (5'-TCCGTAGGTGAACCTGCGG-3') and ITS4 (5'-TCCTCGCTTATTGATATGC-3') (34). The detection of fungal fragments was carried out with the fluorescent dye EvaGreen (Biotium, San Francisco, California, USA). Amplifications were performed in 50 μL reaction volume as described in Vogel et al. (32) with the modification of 4% DMSO. The serially diluted gel-purified ITS fragment from Phomopsis sp. cloned into pGEM-T vector was used for generating standard curves (technical triplicates; average efficiency = 81.4%; R2 = 0.998). The specificity of EvaGreen detection was checked by melting curve analysis. Amplifications and detections were performed in the Thermocycler CFX96 Real Time PCR System (Bio-Rad Laboratories, Inc., Hercules, California, USA). Logarithmic transformed data was related to the soil dry weight.
16S rRNA Gene and ITS2 Fragment Amplicon Sequencing
As described in Fernandez-Gnecco et al. (12), amplicon sequencing libraries were prepared using a two-step PCR targeting the V3-V4 region of the bacterial and archaeal 16S rRNA gene or the ITS2 region for fungal community profiling. Briefly, the V3-V4 region of the 16S rRNA gene was amplified using primers Uni341F (5'-CCTAYGGGRBGCASCAG-3') and Uni806R (5'- GGACTACHVGGGTWTCTAAT-3') (35–37) with PCR conditions as described in Babin et al. (21). The first ITS2 PCR was performed with the primers gITS7 (5'-GTGARTCATCGARTCTTTG-3') and ITS4 (5'-TCC TCC GCT TAT TGA TAT GC-3') (38) with PCR conditions as described in Fernandez-Gnecco et al. (12). PCR products were checked by 1% agarose gel electrophoresis using 0.5X TBE buffer and stained with 0.005% ethidium bromide.
Illumina sequencing adapters and sample-specific dual indexes (IDT Integrated DNA Technologies, Coralville, Iowa, USA) were added in a second PCR using PCRBIO HiFi (PCR Biosystems Ltd., London, UK) for 15 amplification cycles. As for the first PCR, amplification products were purified using HighPrep PCR clean-up (MagBio Genomics, Gaithersburg, USA, ratio 0.65:1). SequalPrep Normalization Plate (96) Kits were used to normalize sample concentrations (Thermo Fisher Scientific, Waltham, Massachusetts, USA). The libraries were then pooled and concentrated using DNA Clean and Concentrator-5 Kit (Zymo Research, Irvine, California, USA). After determining 16S rRNA gene and ITS2 pool concentrations using the Quant-iT High-Sensitivity DNA Assay Kit (Life Technologies, Carlsbad, California, USA), the libraries were denatured and diluted to 8 pM. Sequencing was performed on an Illumina MiSeq platform using Reagent Kit v2 (2 × 250 cycles; Illumina, San Diego, California, USA) following the manufacturer's instructions.
Sequence Analysis
Cutadapt version 2.3 (39) was used to remove primer sequences from first PCR, and only read pairs containing both primer sequences were kept. Reads were further processed for error correction, merging, and generation of amplicon sequence variants (ASVs) using DADA2 version 1.10.0 (40) plugin for QIIME2 (41) with the following parameters: truncL = 0, truncR = 0; trimL = 8, trimR = 8, a minimum overlap of five nucleotides, and otherwise default parameters. Each ASV was taxonomically annotated using q2-feature-classifier classify-sklearn module trained with SILVA SSU rel. 132 database (42), trimmed for the V3-V4 region for bacterial and archaeal community analysis or with the untrimmed UNITE database v7.2 dynamic (43) for fungal community analysis.
Singletons, potential contaminants based on the negative control, and non-target reads (chloroplasts and mitochondria for 16S rRNA gene data) were removed. Additionally, to account for PCR and sequencing artifacts, microbial ASVs with fewer than five reads across the full data set were excluded from further analyses. For 16S sequencing data, curation consisted of ambiguous taxonomy renaming. Decontam R package (44) was used to filter ASVs identified as PCR contaminants using the “prevalence” method. Only for ITS data, a potential contaminant ASV, identified as Penicillium sp., was found and removed in winter samples, which represented up to 51% of reads. The resulting final number of ASVs and quality-filtered reads per sample and treatment can be found in Supplementary Tables 1–3. For 16S rRNA gene data, the cleaning resulted in a final number of 3,814 ASVs and 421,991 high-quality reads for the winter sampling and 4,970 ASVs and 519,482 reads for the summer sampling. For ITS data, a total of 175 ASVs and 85,081 reads for the winter sampling and 885 ASVs and 1,516,993 reads for the summer sampling were obtained.
Statistical Data Analysis
According to the experimental design, tillage practice and N-fertilization intensity were treated as fixed factors while sampling time was considered random. Therefore, for each sampling time separately, a two-factorial model was used for the statistical analysis of soil chemical characteristics, microbial gene copy numbers, and alpha- and beta-diversity metrics. Soil chemical characteristics, alpha-diversity indices and qPCR results were subjected to analysis of variance (ANOVA) after checking whether data meets ANOVA assumptions (Shapiro-Wilk's and Levene's tests). When ANOVA assumptions failed, data was log10 transformed to achieve a Gaussian distribution. To conduct comparison between two individual treatments (CT.Ext vs. CT.Int, MP.Ext vs. MP.Int, CT.Ext vs. MP.Ext, and CT.Int vs. MP.Int), Student's t-test was performed. Alpha-diversity indices (Species richness, Shannon diversity, and Pielou's evenness) were calculated per sample based on 100 times randomly to the least number of sequences per data set (bacterial and archaeal community: winter n = 13,905 or summer n = 3,962 reads; fungal community: winter n = 3,460 or summer n = 65,376 reads) subsampled read count data. In order to test the effect of tillage and N-fertilization intensity on the microbial community composition (beta-diversity), a permutational multivariate analysis of variance [PERMANOVA; (45)] was used. The PERMANOVA analysis was based on Bray–Curtis dissimilarity matrices using 10,000 permutations calculated from logarithmic transformed data. Differences among community compositions were visualized using non-metric multidimensional scaling (NMDS) and constrained analysis of principal coordinates (CAP). Both methods were based on Bray–Curtis dissimilarities and were performed with logarithmic transformed data. To correlate microbial community composition with soil chemical parameters, the function envfit (package vegan) was used.
To test for microbial genera with significantly different relative abundance between CT vs. MP or Int vs. Ext, respectively, a likelihood ratio test under negative binomial distribution and generalized linear models (FDR-corrected p < 0.05) was carried out separately per sampling time and for each N-fertilization intensity or tillage type, respectively. The normalization of count data was performed using correction factors for the library size as recommended by the developers (edgeR), and only genera present in more than three samples across the data set were considered (relative abundance > 0.5%). To graphically display the relative abundance distribution of the most abundant genera affected by agricultural practices, a heat map based on relative abundances for each taxon was drawn (horizontal clustering based on Euclidean distance). For each sampling time, a Venn diagram was generated to compare the presence of ASVs in the different agricultural practices and to identify the core microbiota, i.e., ASVs present in all treatments. Fungal trophic modes at genus level were predicted using the software tool FUNGuild following developers' recommendations (46).
All analyses were carried out with RStudio version 3.6.1 (https://www.r-project.org/) using the following R packages: vegan (47), agricolae (48), rcompanion (49), car (50), edgeR (51), ggplot2 (52), rioja (53), phyloseq (54), labdsv (55), mvabund (56), pheatmap (57), BiocManager (58), and VennDiagram (59).
Results
Soil Chemical Properties
Observed effects of tillage practice on soil chemical properties were independent of the sampling time. Two-way ANOVA showed that TN, TC, TOC, and available K and P as well as pH (all p < 0.001) were significantly affected by the tillage practice. Pairwise comparisons showed that the amount of TN, TC, TOC, and available K and P were significantly higher in CT compared with MP irrespective of the N-fertilization intensity while pH showed the opposite trend (7.5 or 7.3 for MP or CT, respectively; Table 2). Regarding N-fertilization intensity, effects on soil chemical properties depended on the sampling time. Two-way ANOVA showed that the N-fertilization intensity significantly affected the amount of available K (p = 0.001) in soils sampled in winter while TC content and available P levels (both p < 0.001) were affected by N-fertilization intensity in soils sampled in summer. Pairwise comparisons indicated higher levels of available K in CT.Ext vs. CT.Int in soils sampled in winter and higher TC content in MP.Int vs. MP.Ext and higher available P levels in MP.Ext vs. MP.Int in soils sampled in summer. Other parameters (pH, TOC, TN) were not affected by N-fertilization intensity.
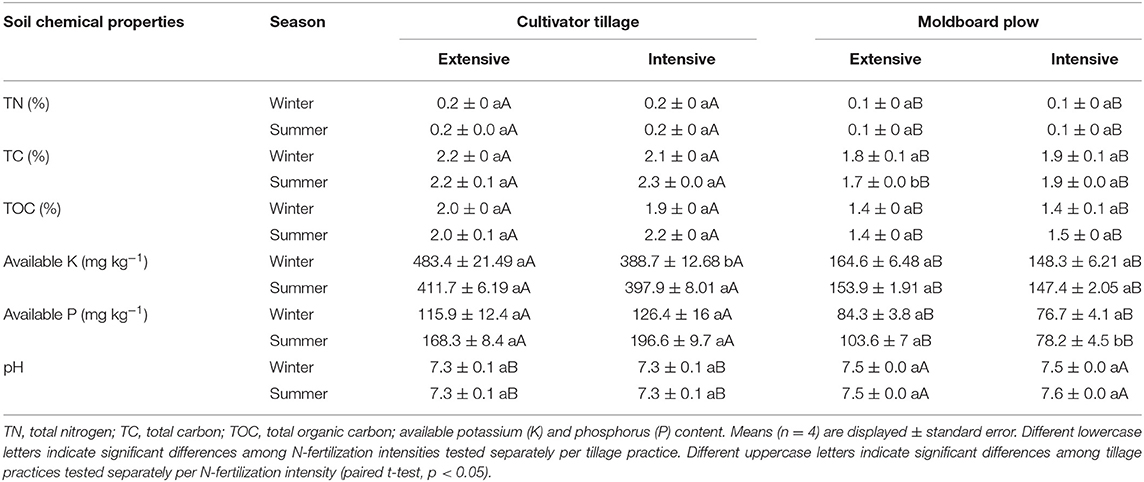
Table 2. Chemical properties in soils under different long-term tillage practices (cultivator tillage vs. moldboard plow) and N-fertilization intensities (intensive vs. extensive) in LTE Bernburg.
Quantification of Bacterial and Fungal Markers
Two-way ANOVA showed that the 16S rRNA gene copy numbers were in both sampling times affected by neither tillage practice nor N-fertilization intensity. Bacterial 16S rRNA gene copy numbers ranged in winter from 9 × 108 to 1 × 109 and in summer from 7 × 108 to 1 × 109 per gram of dry soil (Supplementary Figure 1A).
In contrast, two-way ANOVA showed that ITS fragment copy numbers were significantly affected by the tillage practice in winter (p = 0.001) and summer (p = 0.004) but not by N-fertilization intensity. Fungal ITS copy numbers ranged in winter from 1 × 107 to 1 × 108 and in summer from 6 × 106 to 1 × 107 per gram of dry soil (Supplementary Figure 1B). Pairwise comparisons showed that soils from CT exhibited higher ITS copy numbers compared with MP under both N-fertilization intensities (Ext and Int) in winter, whereas in summer, this observation was only made in Int.
Soil Microbial Alpha-Diversity Patterns
Rarefaction curves (Supplementary Figure 2) showed that the sequencing depth was sufficient to cover the microbial diversity present in each sample.
Two-way ANOVA revealed that both tillage practice and N-fertilization intensity significantly affected the alpha-diversity of bacterial and archaeal communities in soils sampled in winter (Species richness and Shannon diversity, all p < 0.001; Figures 1A,B). Diversity estimators showed higher diversity in MP vs. CT and in Int vs. Ext. Pielou's evenness did not differ between Int vs. Ext in winter but was significantly higher in CT vs. MP (p < 0.001). Bacterial and archaeal Species richness and Shannon diversity in soils sampled in winter were significantly higher in MP.Int compared with CT.Int (Figures 1A,B) but the opposite was observed for Pielou's diversity (Figure 1C). In soils sampled in summer, two-way ANOVA revealed that both, tillage practice and N-fertilization intensity, affected the alpha-diversity of bacterial and archaeal communities (Species richness and Shannon diversity, all p < 0.01), resulting in higher bacterial/archaeal diversity in CT vs. MP. Pielou's evenness was only affected by tillage practice (p = 0.02), resulting in higher bacterial/archaeal diversity in Ext vs. Int. Contrary to winter, diversity estimators (Species richness, Shannon diversity) for bacterial and archaeal communities in soils sampled in summer from CT.Int had a significantly higher alpha-diversity compared with MP.Int, while no significant differences among individual treatments were observed for Pielou's evenness (Figure 1C).
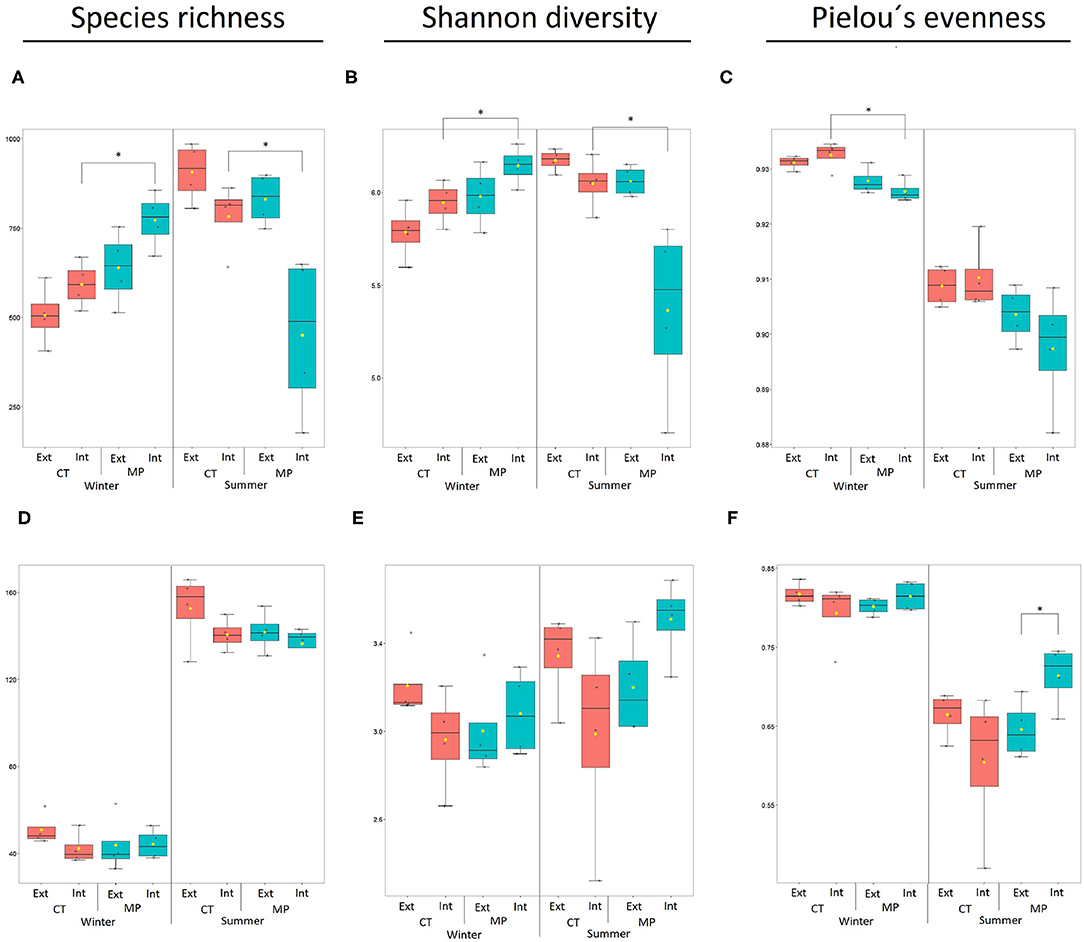
Figure 1. Diversity indices of bacterial/archaeal (A–C) and fungal (D–F) communities in soils sampled in LTE Bernburg in winter or summer under different long-term tillage practices (CT, Cultivator Tillage vs. MP, Moldboard Plow) and N-fertilization intensities (Int, intensive vs. Ext, extensive). Boxplots show the mean (yellow dot), min./max. (whiskers), and replicates per sample (n = 4; black dots). Asterisk indicates significant differences between treatments (paired t-test, p < 0.05).
Regarding the alpha-diversity of fungal communities in soils sampled in winter, two-way ANOVA revealed that diversity estimators (Species richness, Shannon diversity, and Pielou's evenness) were affected by neither tillage practice nor N-fertilization intensity. In soils sampled in summer, two-way ANOVA revealed a significant interaction between tillage practice and N-fertilization intensity on fungal Shannon diversity and Pielou's evenness (both p = 0.04), resulting in higher indices in CT.Ext vs. MP.Ext and MP.Int vs. CT.Int treatments. Pairwise comparisons showed that the Pielou estimator was higher in MP.Int than in MP.Ext in soils sampled in summer (Figures 1D–F).
Soil Microbial Beta-Diversity Patterns
Soil microbial communities under different tillage practice and N-fertilization intensity were analyzed by PERMANOVA, which showed that both the bacterial/archaeal and fungal community composition in soils sampled in winter were significantly affected by tillage practice but not by N-fertilization intensity (Table 3). In summer, both factors significantly affected the microbial community composition with tillage being a stronger driver than N-fertilization intensity.
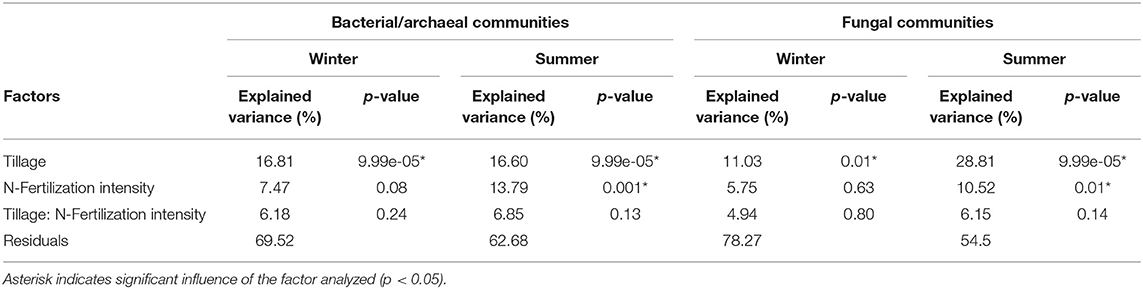
Table 3. Effect of long-term tillage practice and N-fertilization intensity on soil microbial communities in LTE Bernburg.
NMDS ordination showed a clear tillage-dependent clustering at both sampling times for bacterial/archaeal communities (Supplementary Figures 3A,B). Fungal communities were grouped by tillage practice with subclusters corresponding to the N-fertilization intensity in summer, but no treatment-dependent clustering was observed in winter (Supplementary Figures 3C,D).
CAP analysis confirmed PERMANOVA results for both 16S rRNA gene (Figures 2A,B) and ITS data (Figures 2C,D). Tillage practice shaped the microbial community composition, resulting in a distinct clustering of MP and CT samples at both sampling times. Microbial communities in CT soils were significantly positively correlated with P (except for ITS data in winter), K, TOC, TN, and TC content, whereas microbial communities in MP soils were significantly positively correlated with the soil pH (all p < 0.01), independently of the sampling time.
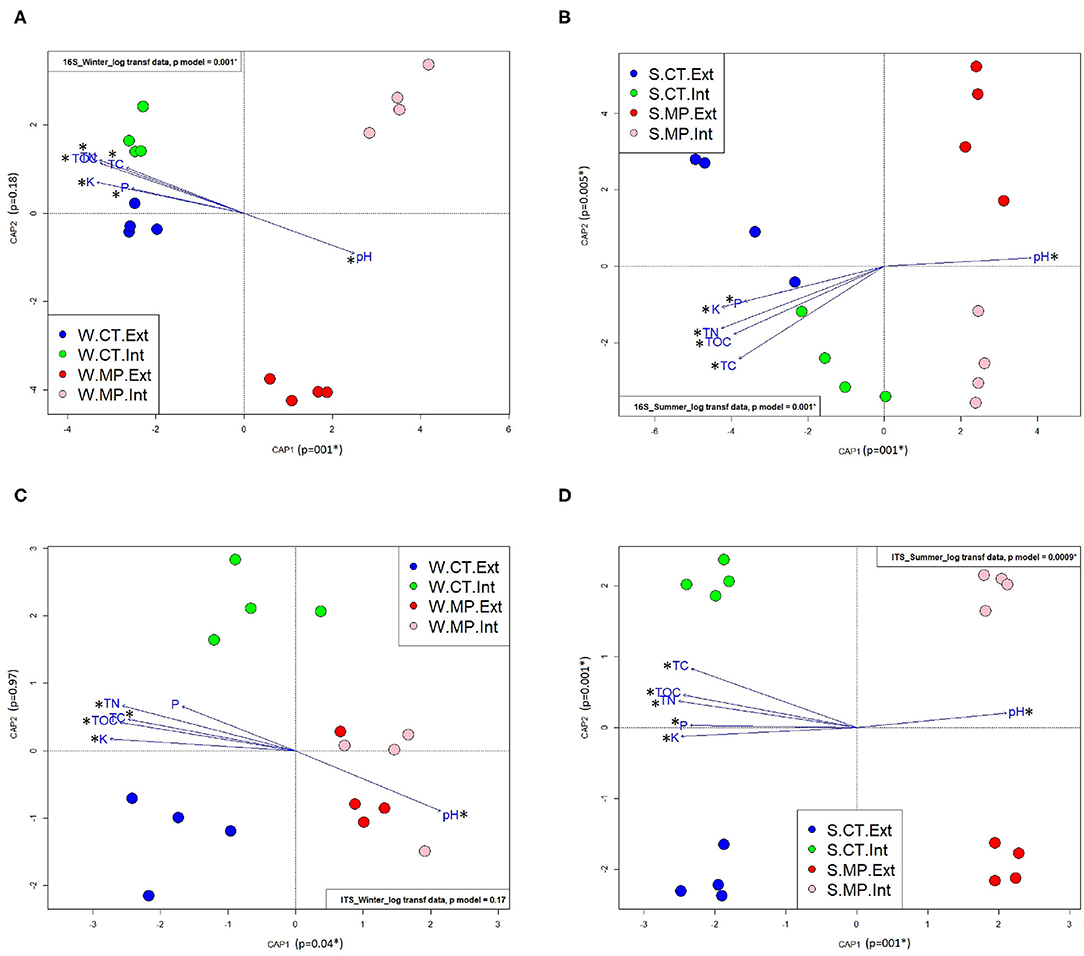
Figure 2. Constrained analysis of principal coordinates (CAP) using Bray–Curtis distance of bacterial/archaeal (A,B) and fungal (C,D) communities in soils sampled in LTE Bernburg in winter (W) or summer (S) under different long-term tillage practices (CT, Cultivator Tillage vs. MP, Moldboard Plow) and N-fertilization intensities (Int, intensive vs. Ext, extensive). Asterisks indicate a significant correlation of the chemical parameter with the community composition (p < 0.05). Soil chemical parameters: total nitrogen (TN), total carbon (TC), total organic carbon (TOC), available potassium (K), and phosphorus (P) content and pH.
Effects of Tillage and N-Fertilization Intensity on Soil Bacterial/Archaeal Taxa
The taxonomic composition at phylum level (Supplementary Figure 4A) was dominated by Bacteroidetes (9 or 37%, winter or summer, respectively), Proteobacteria (25 or 26%), Acidobacteria (17 or 8%), Actinobacteria (23 or 0.5%), Thaumarchaeota (12 or 9%), and Firmicutes (3 or 7%). In winter, most of the differences in phylum relative abundance among the treatments were due to the tillage practice, resulting in a significantly higher relative abundance of Actinobacteria (p = 0.002) in CT compared with MP. In summer, in contrast, the phylum Firmicutes exhibited a significantly higher relative abundance (p = 0.01) in MP soils compared with CT. Furthermore, also the N-fertilization intensity affected the bacterial/archaeal phylum composition in summer. For instance, Proteobacteria (p = 0.007), Acidobacteria (p = 0.04), Actinobacteria (p = 0.01), and Thaumarchaeota (p = 0.005) exhibited higher relative abundances in Int vs. Ext treatments, whereas Bacteroidetes showed the opposite trend (p = 0.01).
At the genus level, in total, 592 bacterial/archaeal genera were detected, and sequences with closest affiliation to Nitrososphaeraceae (Thaumarchaeota; 11 or 9%, winter or summer, respectively), Microscillaceae (Bacteroidetes; 1 or 8%), Chitinophagaceae (Bacteroidetes; 1 or 7%), Bacillus (Firmicutes; 2 or 5%), and Sphingomonas (Proteobacteria; 3 or 4%) were predominant.
In winter, several bacterial (but not archaeal) genera with significantly different relative abundances in MP vs. CT were identified (Supplementary Table 4; Figure 3), whereas no N-fertilization intensity-dependent genera were detected (Supplementary Table 5; Figure 3). For instance, acidobacterial taxa (RB41 and Subgroup 7) had significantly higher relative abundances in MP treatments and actinobacterial genera (Rubrobacter and Nocardioides) in CT treatments, irrespective of the N-fertilization intensity. Additionally, Microvirga (Proteobacteria) had a higher relative abundance in CT.Int compared with MP.Int, and sequences with the closest affiliation to Gemmatimonadaceae (Gemmatimonadetes) were higher in MP.Ext compared with CT.Ext indicating interaction effects between tillage and N-fertilization intensity.
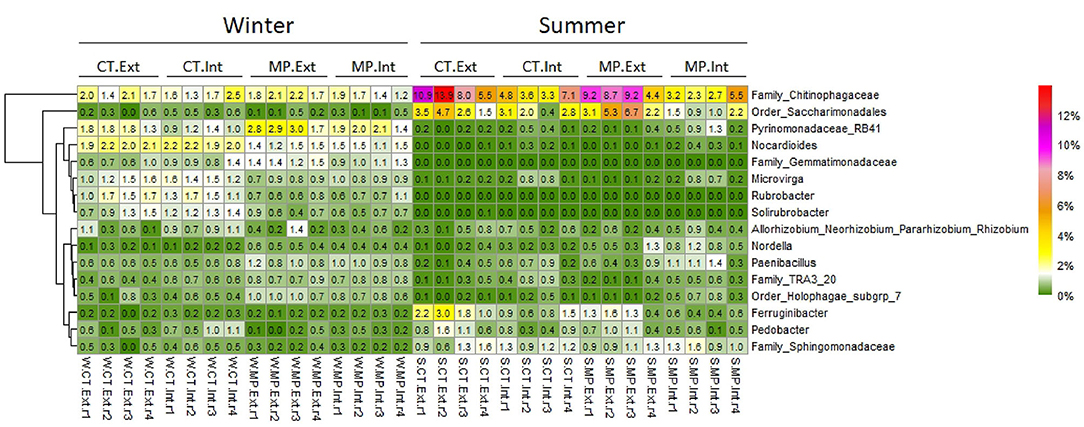
Figure 3. Heatmap displaying the relative abundance distribution of bacterial genera (responder) significantly affected (FDR < 0.05) by different long-term management practices (CT, Cultivator Tillage vs. MP, Moldboard Plow; Int, intensive vs. Ext, extensive) in soils sampled in LTE Bernburg in winter (W) or summer (S). Most abundant responders with average (n = 4) relative abundance per treatment >0.5% are shown. Pairwise comparisons between treatments are presented in Supplementary Tables 4, 5.
In contrast, in summer, many genera with significantly different relative abundances between N-fertilization intensities were detected, and only a few genera (under 1% of relative abundance) were found to differ in relative abundance depending on tillage practice (Figure 3; Supplementary Tables 4, 5). For instance, Ferruginibacter and sequences with the closest affiliation to Chitinophagaceae (both belonging to the phylum Bacteroidetes) were significantly enriched under Ext compared with Int independent of the tillage practice. In MP soils, a significantly higher relative abundance of sequences with the closest affiliation to Saccharimonadales (Patescibacteria) was observed in Ext compared with Int.
Effects of Tillage and N-Fertilization Intensity on Soil Fungal Taxa
The taxonomic composition at the phylum level (Supplementary Figure 4B) was dominated by the phylum Ascomycota (51 or 57%, winter or summer, respectively), Basidiomycota (33 or 12%), Mucoromycota (1 or 22%), Mortierellomycota (13 or 6%), Chytridiomycota (0.8 or 1%), and Glomeromycota (0.05 or 0.3%). At both sampling times, the phylum Mucoromycota exhibited a significantly higher relative abundance in CT compared with MP while Glomeromycota showed the opposite trend (both p < 0.02). In summer, Basidiomycota had a higher relative abundance in MP compared with CT (p = 0.01).
At genus level, 241 fungal genera were detected, among which Exophiala (Ascomycota, 11 or 16%, winter or summer, respectively), Rhizopus (Mucoromycota, 2 or 22%), Bolbitius (Basidiomycota, 20 or 0.05%), and Mortierella (Mortierellomycota, 13 or 6%) were the most abundant.
In order to obtain further insights into the ecological assignment of detected fungal genera, a tentative classification into pathotrophic, saprotrophic or symbiotrophic, or multiple trophic modes was made using FUNGuild (Supplementary Figure 5). Circa 77% of fungal reads could be classified at the genus level. In soils sampled in winter, fungal trophic modes were affected by tillage practice and the interaction with N-fertilization intensity, resulting in the highest relative abundance of saprotrophic fungi in CT.Ext (p = 0.03). In soils sampled in summer, the fungal trophic assignment was influenced by N-fertilization intensity and the interaction with tillage practice, resulting in the lowest relative abundance of genera classified as pathotroph-saprotroph in MP.Int (p = 0.03).
The analysis of differentially abundant fungal genera among treatments showed that, in soils sampled in winter, the genus Rhizopus (classified as pathotroph-saprotroph-symbiotroph, Mucoromycota) was most strongly affected by tillage practice, displaying higher relative abundances in CT than MP (Figure 4; Supplementary Table 6). No fungal genera significantly responding to N-fertilization intensity were detected in winter (Supplementary Table 7). In soils sampled in summer, differentially abundant fungal taxa were found to be affected by tillage practice and N-fertilization intensity. For instance, the genus Gibberella (pathotroph; Ascomycota) showed a significantly higher relative abundance in CT compared with MP. Metarhizium (pathotroph-symbiotroph; Ascomycota) was enriched under CT.Ext compared with MP.Ext (Supplementary Table 6). Minimedusa (no trophic mode assigned; Basidiomycota) and Gibellulopsis (pathotroph; Ascomycota) were significantly enriched under Int compared with Ext. Soils under MP.Int exhibited a higher relative abundance of the genus Exophiala (pathotroph-symbiotroph; Ascomycota) compared with MP.Ext, whereas Penicillium (no trophic mode assigned; Ascomycota) showed the opposite trend. Genus Rhizopus showed different responses to N-fertilization intensity depending on the tillage type (higher relative abundance in CT.Int vs. CT.Ext but higher in MP.Ext vs. MP.Int; Figure 4; Supplementary Table 7).
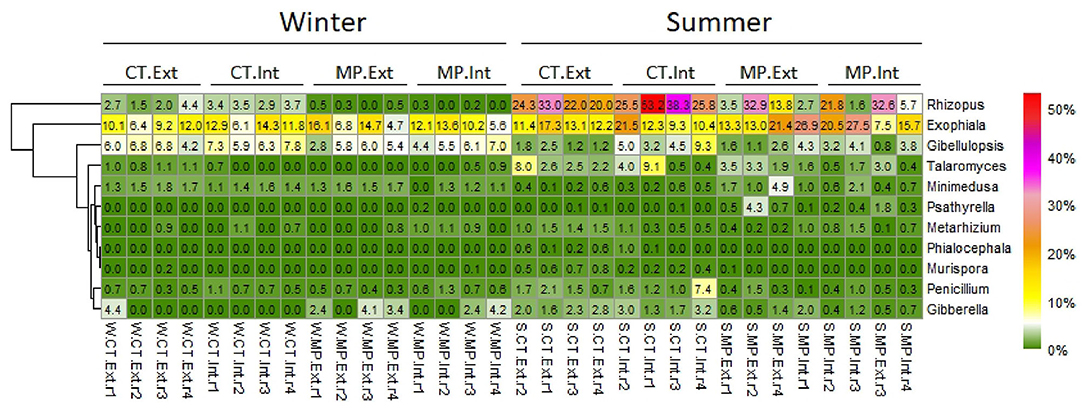
Figure 4. Heatmap displaying the relative abundance distribution of fungal genera (responder) significantly affected (FDR < 0.05) by different long-term management practices (CT, Cultivator Tillage vs. MP, Moldboard Plow; Int, intensive vs. Ext, extensive) in soils sampled in LTE Bernburg in winter (W) or summer (S). Most abundant responders with average (n = 4) relative abundance >0.5% are shown. Pairwise comparisons between treatments with assignment into functional guilds are presented in Supplementary Tables 6, 7.
Soil Bacterial/Archaeal Core Microbiota in the LTE Across Different Agricultural Practices
Regardless of tillage practice or N-fertilization intensity, a soil core microbiota, defined here as ASVs present in soils of all four investigated treatments (CT.Ext, CT.Int, MP.Ext, and MP.Int), was detected at each sampling time. The winter bacterial/archaeal core microbiota consisted of 484 ASVs (Figure 5A), representing 13% of the total ASVs detected in all soils. Most of these bacterial/archaeal core ASVs were affiliated to the phyla Actinobacteria, Proteobacteria, and Acidobacteria (all ca. 25%; Figure 5C). Classification at lower taxonomic levels revealed RB41 (Acidobacteria; 2%) and unclassified genera of Micrococcaceae (3%) and Deltaproteobacteria (3%) as major core bacteria. The summer core microbiota consisted of 555 ASVs (Figure 5B), representing 12% of the total ASVs. Among all summer core ASVs, 35% were affiliated to the phylum Bacteroidetes, 29% to Proteobacteria and 10% to Acidobacteria (Figure 5D). Most of these ASVs belonged to unclassified genera of the families Microscillaceae (8%), Methylophilaceae (1%), and Blastocatellaceae (3%).
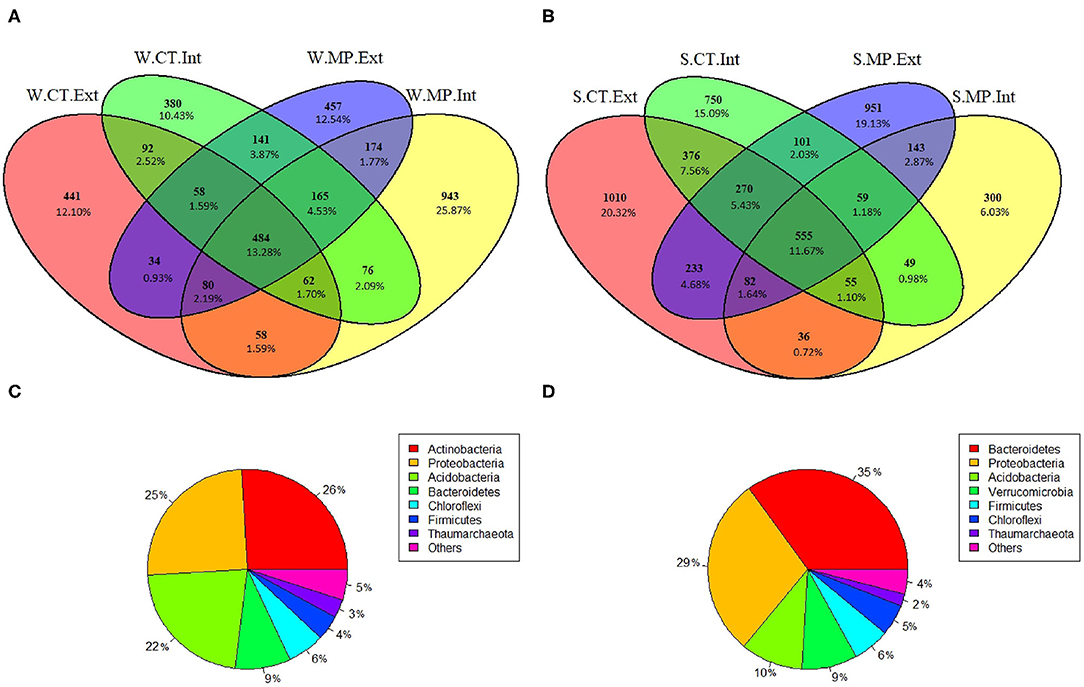
Figure 5. Venn Diagrams (A,B) showing unique and shared bacterial/archaeal ASVs among soils sampled in LTE Bernburg in winter (W) or summer (S) under different long-term tillage practices (CT, Cultivator Tillage vs. MP, Moldboard Plow) and N-fertilization intensities (Int, intensive vs. Ext, extensive). Taxonomic classification (C,D) at the phylum level of ASVs shared among all four treatments in winter or summer, respectively.
The core microbiota shared between winter and summer comprised 177 ASVs (data not shown). These sampling time-independent ASVs were mainly affiliated to Chitinophagaceae (13%), Acidobacteria_subgrp_6 (7%), and Sphingomonadaceae (5%).
Soil Fungal Core Microbiota in the LTE Across Different Agricultural Practices
Regardless of tillage practice or N-fertilization intensity, a winter fungal core microbiota consisting of 45 ASVs was identified (Figure 6A), representing 26% of the total ASVs. Most of the core ASVs belonged to the phyla Ascomycota (58%) and Basidiomycota (29%) (Figure 6C). At lower taxonomic levels, fungal core ASVs at the winter sampling were classified mainly as genera Bolbitius (20%) and Exophiala (10%). The summer core microbiota consisted of 125 ASVs (Figure 6B), representing 15% of the total ASVs. The phyla Ascomycota (63%) and Basidiomycota (21%) were again most represented among the core ASVs (Figure 6D). At lower taxonomic levels, most of the fungal core ASVs at the summer sampling were affiliated to the genera Exophiala (16%) and Solicoccozyma (3%).
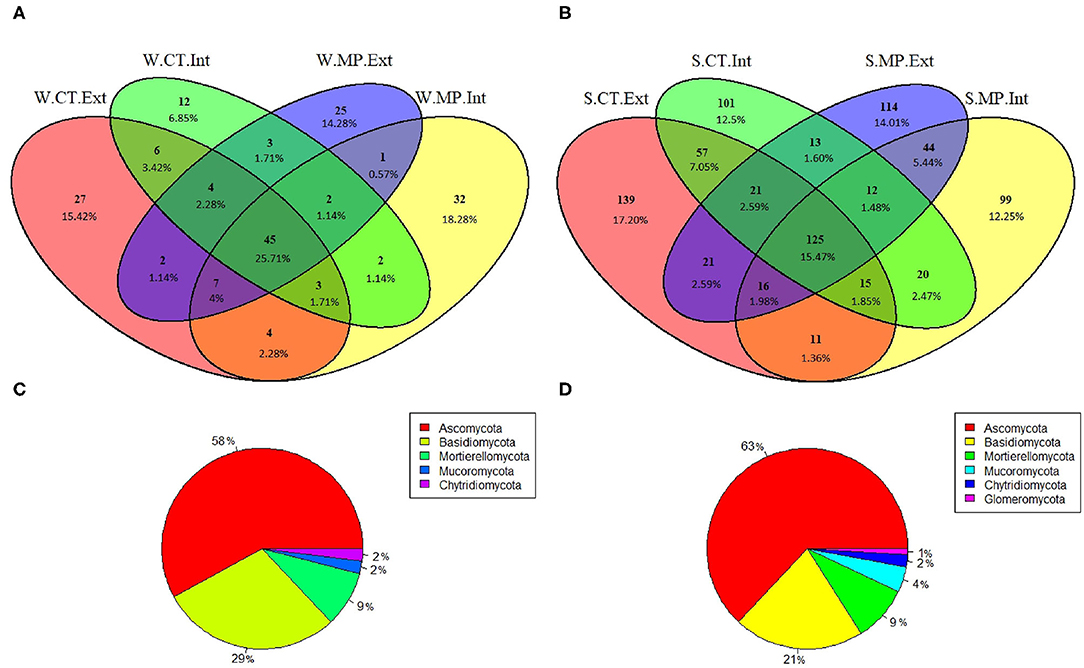
Figure 6. Venn Diagrams (A,B) showing unique and shared fungal ASVs among treatments in soils sampled in LTE Bernburg in winter (W) or summer (S) under different long-term tillage practices (CT, Cultivator Tillage vs. MP, Moldboard Plow) and N-fertilization intensities (Int, intensive vs. Ext, extensive). Taxonomic classification (C,D) at phylum level of shared ASVs in winter or summer, respectively.
The comparison between fungal core microbiota in winter and summer showed 21 common ASVs (data not shown), which were mainly affiliated to the genera Mortierella (15%), Bolbitius (5%), and Exophiala (5%).
Discussion
In this study, we aimed to determine to what extent long-term intensive and conserved agricultural management practices (tillage practice and N-fertilization intensity) affect soil chemical and microbial parameters depending on the sampling time. Soil samples were taken in absence of plants in November (winter; two weeks after tillage) and in the following growing season in July in presence of maize (summer; one month after N-fertilization).
We observed that agricultural management shaped the soil microbiota, but the effects detected were sampling time-dependent. Tillage practice was the main driver of the soil microbiota in winter while, in summer, also the N-fertilization intensity exerted a strong effect (alpha- and beta-diversity, responder analysis). This confirms our first hypothesis and is likely a direct short-term response to the applied agricultural management. The studied model crop was maize, which does not require vernalization. Therefore, the winter sampling was carried out in fallow soils shortly after soil tillage while the summer sampling took place in presence of maize under the influence of respective fertilization/plant protection measures. Agricultural practices such as crop rotation, periodic fertilization, and pesticide use result in temporal and spatial changes in soil chemical properties and, therefore, in differences in nutrient availability for microorganisms (60). It has been shown previously that different long-term tillage practices shape the soil microbial community structure (20, 21, 61). Tillage is known to make protected OM available for microbial degradation (62). Furthermore, tillage results in a destruction and transformation of microhabitats by mechanical breakup of soil aggregates (niche condition homogenization) and dislocation of the soil microbiota along the soil profile (63).
Apart from these short-term responses, our results support a legacy management effect in summer (several months after MP or CT application) suggesting that the soil disturbance had a long-lasting influence contrary to N-fertilization intensity. Schlüter et al. (64) showed for the same LTE that tillage changed the soil structure and hydraulic properties in the long term. This legacy likely promotes different conditions for microbial survival and growth and, therefore, resulted in the observed long-lasting effect on the soil microbiota. Therefore, the legacy of agricultural practices needs to be considered as an additional variable in plant-soil feedback loops (26, 27).
Contrary to our results obtained from the summer sampling, previous studies in the LTE show that N-fertilization intensity had little or no effect on the soil microbial community (18, 20, 21). In the LTE, mineral N-fertilization is performed when the crop starts growing (for maize: in spring). In comparison to Sommermann et al. (20) and Babin et al. (21), who sampled soils from winter wheat fields in the late generative phase at harvest (July), where roots are largely inactive, we analyzed an earlier time point of the maize growing cycle during vegetative growth, characterized by high root activity and nutrient uptake (65). Particularly, during vegetative growth with intense nutrient uptake, different fertilization intensity can influence the type and extension of root-induced changes in the nearby soil chemistry and the composition and quantity of rhizodeposits with potential feedback loops on rhizosphere microbial communities. However, it should be kept in mind that the observed N-fertilization intensity effect on the soil microbiota at the summer sampling could be caused by the different levels of N-fertilization or long-term fungicide use. Mineral N fertilizer (urea and ammonia) are known to affect soil pH (66). Although no differences in soil pH in Int vs. Ext were detected here, temporal effects shortly after fertilizer application cannot be excluded. Furthermore, the high N inputs in Int could have inhibited some soil microorganisms (66). The long-term fungicide use in Int might have had direct negative effects on the fungal communities in these soils.
Only a small proportion of the detected ASVs was permanently detected in the soils, i.e., independent of the sampling time and management. This core microbiota was constituted of taxa typically associated with agricultural soils (e.g., Sphingomonadaceae, Chitinophagaceae, Mortierella, Bolbitius, and Exophiala). Sphingomonadaceae, Chitinophagaceae, and Mortierella were previously detected in the LTE soils (20, 21). This indicates that these taxa are specific for the soil site and are not responding to physicochemical changes caused by the agricultural management. At the different sampling times, a different core microbiota was detected. This shows that, besides dynamics in the soil microbial community compositions due to agricultural practices, there is also a season-dependent succession in the composition of the soil microbial communities.
For both sampling times, distinct soil microbial communities were observed in CT and MP. This was in concordance with previous studies in the LTE (18, 20, 21). Differences in the microbiota are likely linked to the observed tillage-dependent differences in soil chemical parameters. In this study, we could show that the soil microbial community composition under CT was strongly positively correlated with soil nutrients (e.g., K, P, TOC, and TC) but negatively correlated with pH. In fact, it was shown previously that CT changes the labile carbon pool in the soil (67). Soils under long-term reduced tillage with residues remaining on the soil surface promote soil stratification as observed for CT in the LTE, e.g., in terms of available P and K (28). Our data suggest that the higher TOC content in CT compared with MP in the topsoil layer (0–20 cm) promoted fungal growth and the enrichment of saprotrophs. Consistently, we observed higher fungal ITS fragment copy numbers in CT vs. MP. Soils under CT.Ext exhibited also a higher relative abundance of predicted saprotrophs in winter in comparison with MP.Ext. The saprotrophic fungus Rhizopus (Mucoromycota) had a higher relative abundance in soils under CT practice independent of the sampling time. Members of this fungal genus produce a variety of enzymes that enable them to utilize a wide range of nutrients and, therefore, to play a key role in the decomposition of organic materials (68, 69). Since plant residues are accumulated in the topsoil under CT, we, therefore, suggest that Rhizopus had a competitive advantage over other saprotrophs and established in the long term. This is in agreement with Srour et al. (61) who show that the accumulation of crop residues on the surface resulted in an increase in soil OM in the top layer promoting the proliferation of obligate saprotrophic fungi. In agreement with Babin et al. (21), acidobacterial taxa (such as RB41, Subgroup 7) had higher relative abundances in the MP treatment in comparison with CT. It is reported that a high relative abundance of Acidobacteria is indicative for oligotrophic soils with lower C and nutrient levels (70). The enrichment of actinobacterial genera, which are able to degrade complex organic compounds (22) in CT treatments in comparison with conventional MP tillage was likely related to the decomposition of crop residues remaining on the soil surface. As the decomposition of OM plays a critical role in the supply of crops with nutrients (13), we propose that CT fosters soil fertility.
Furthermore, CT and MP soils differed in the relative abundance of potential plant beneficial and pathogenic microorganisms. For instance, the relative abundance of the putative beneficial pathotroph-symbiotroph fungi Metarhizium (phylum Ascomycota) was significantly increased under CT tillage practice. Members of the genus Metarhizium are reported to have plant growth-promoting traits as well as biological control activity against insect pathogens (71, 72). The fact that the plant beneficial symbiotic AMF exhibited highest relative abundance in soils under MP tillage could be linked with the lower available P level found in these treatments in comparison with CT. This is in accordance with previous reports that stated that AMF are negatively affected (soil diversity and root colonization) by high available P levels in soils (73, 74). In addition, plow tillage can influence AMF activity by disrupting hyphal networks causing dispersion of propagules (75). Soils under CT practice also showed a high relative abundance of Gibberella in summer irrespective of the N-fertilization intensity. Sommermann et al. (20) reported that the relative abundance of Gibberella/Fusarium was enriched in CT.Int soils cultivated with wheat after maize in the LTE. Members of Gibberella are known plant pathogens, such as Gibberella zeae (anamorph = Fusarium graminearum Schwabe), which causes a mycotoxin contamination in maize, the so-called “ear rot” disease. The pathogen has the ability to survive in crop residues on the soil surface (76, 77), which presents a risk of managing soils by CT.
Irrespective of tillage practice, soils under Ext fertilization exhibited high relative abundances of sequences with the closest affiliation to Chitinophagaceae and the genus Ferruginibacter (both phylum Bacteroidetes). Members of the Bacteroidetes phylum were reported to have plant-beneficial characteristics (78). Additionally, Bacteroidetes species have the ability to degrade complex organic compounds, such as fungal cell walls (79), which means that they can act as antagonists toward fungal pathogens. Soils under Int fertilization showed a high relative abundance of Gibellulopsis in summer irrespective of the tillage practice. This genus contains saprophytes and opportunistic plant pathogens, such as Gibellulopsis nigrescens [basionym: Verticillium nigrescens Pethybridge (80)], which causes vascular wilt diseases in numerous hosts and can survive in soil or on dead plant material (81). However, also the potential beneficial genus Minimedusa, which was reported as antagonistic toward Fusarium sp. (82), was higher under Int fertilization practice. Based on metagenomic analysis, Srour et al. (61) revealed that long-term plow tillage and intensive N-fertilization management shifted soil microbial communities toward fast-growing competitors (such as pathogenic species). In the present study, the cosmopolitan fungi Exophiala (predicted as pathotroph-symbiotroph; phylum Ascomycota) showed higher relative abundance in MP.Int vs. MP.Ext. Exophiala has been recently reported in similarly high relative abundance (10–15%) in agricultural soils under intensive management in the Argentinean Humid Pampas (12).
Intensive mineral N-fertilization is reported to be one of the main factors that decreases microbial diversity and number of genera (83). We could confirm this in the present study only for the bacterial/archaeal alpha-diversity in MP.Int soils sampled in summer compared to MP.Ext and contrarily even showed that fungal evenness increased in MP.Int compared to MP.Ext. This suggests that other factors, such as studied organism group, combination with agricultural practices (e.g., long-term fungicide use, tillage practice), and the sampling time point play a role when evaluating the effect of fertilization on the microbial diversity. Therefore, further studies are needed to validate these observations and elucidate the underlying mechanisms.
At the summer sampling time point, significant differences in maize shoot dry mass were recorded (see Supplementary Table 8) with higher dry masses in Ext than Int. This could be due to the abovementioned higher relative abundance of taxa with plant-beneficial characteristics in soils under Ext fertilization. However, this did not influence average grain yields at harvest, which were similarly low in all treatments [4.5 t ha−1 (MT.Ext); 4.6 t ha−1 (CT.Ext); 4.9 t ha−1 (CT.Int); 5.1 t ha−1 (MP.Int)]. The very low maize yields recorded in the growing season under study (2019) compared with previous years was likely due to the low temperature and water deficit that plants faced during early growth (data not shown) resulting in a deficiency of P in plants of all treatments (Supplementary Table 9).
Finally, whether the conserved agricultural practices of CT and Ext have positive effects on soil microbial communities and consequently on soil quality and plant performance depends on various factors. Therefore, further studies under field conditions are needed that consider additional aspects such as local weather, soil type, and the combination with other agricultural practices (e.g., crop rotation).
Concluding Remarks
For the LTE Bernburg, we showed here that the observed responses of the soil microbiota to tillage practices and N-fertilization intensity (with or without growth regulator/fungicide use) differed according to the sampling time. Cultivator tillage (CT) promoted taxa associated with organic matter decomposition resulting in higher nutrient contents, which could foster soil fertility. Moldboard plow (MP) promoted taxa typically associated with oligotrophic environments. Putative beneficial (such as Chitinophagaceae, Ferruginibacter, Minimedusa, and Exophiala) or pathogenic (such as Gibellulopsis and Gibberella) microbial genera were detected responding differently to the agricultural practices. However, further studies considering, e.g., different soil types, crops, and climatic conditions are needed to obtain more insights into how conserved agricultural practices affect plant beneficials and pathogens in soil. Understanding how agricultural management influences soil microbial communities will help to steer the soil microbiota to a desired beneficial state, which can be harnessed for the development of more sustainable agricultural practices and an improved plant performance.
Data Availability Statement
Data sets presented in this study can be found in the NCBI sequence read archive (https://www.ncbi.nlm.nih.gov/). The accession number is PRJNA742917 or PRJNA742938 for 16S rRNA gene or ITS amplicon sequencing datasets, respectively.
Author Contributions
GF-G, DB, FC, VC, and KS contributed to the conception and design of the present research. AD is in charge of the LTE in Bernburg. GF-G performed the experiments and analyzed the data. LM and SS performed the sequencing and contributed to the sequence data analysis. LS, IS, and JG helped with the interpretation of fungal data. JB, NM, GN, and RG analyzed the chemical properties. GF-G wrote the manuscript with DB. All authors contributed to refine the manuscript and approved the final version.
Funding
GF-G was financially supported by the National Scientific and Technical Research Council (CONICET, Argentina) and German Academic Exchange Service (DAAD, Germany). This study was financially supported by DAAD and the German Federal Ministry of Education and Research (BMBF, Germany) within the framework of the project DiControl (http://dicontrol.igzev.de/de/; grant number 031B0514C) as part of the BonaRes funding initiative Soil as a sustainable resource for the bioeconomy (https://www.bonares.de/).
Conflict of Interest
The authors declare that the research was conducted in the absence of any commercial or financial relationships that could be construed as a potential conflict of interest.
Publisher's Note
All claims expressed in this article are solely those of the authors and do not necessarily represent those of their affiliated organizations, or those of the publisher, the editors and the reviewers. Any product that may be evaluated in this article, or claim that may be made by its manufacturer, is not guaranteed or endorsed by the publisher.
Acknowledgments
The present research work is part of the thesis by GF-G in partial fulfillment of the requirements for a Doctor's degree (Facultad de Ciencias Agrarias, Universidad Nacional de Mar del Plata, Argentina). We would like to thank Julian Dege and Gabriel Moyano for their helpful assistance with programming language for data analysis. We would like to thank the Biocomputing Core Facility from the department of Biology at the University of Copenhagen for providing computing power for amplicon analyses.
Supplementary Material
The Supplementary Material for this article can be found online at: https://www.frontiersin.org/articles/10.3389/fsoil.2022.837508/full#supplementary-material
References
1. Foley J, Ramankutty N, Brauman K, Cassidy E, Gerber J, Johnston M, et al. Solutions for a cultivated planet. Nature. (2011) 478:337–42. doi: 10.1038/nature10452
2. Zabel F, Delzeit R, Schneider J, Seppelt R, Mauser W, Václavík T. Global impacts of future cropland expansion and intensification on agricultural markets and biodiversity. Nat Commun. (2019) 10:2844. doi: 10.1038/s41467-019-10775-z
3. Tsiafouli M, Drakou E, Orgiazzi A, Hedlund K, Ritz K. Editorial: optimizing the delivery of multiple ecosystem goods and services in agricultural systems. Front Ecol Evol. (2017) 5:97. doi: 10.3389/fevo.2017.00097
4. Wolfgang A, Taffner J, Guimarães R, Coyne D, Berg G. Novel strategies for soil-borne diseases: exploiting the microbiome and volatile-based mechanisms toward controlling Meloidogyne-based disease complexes. Front Microbiol. (2019) 10:190. doi: 10.3389/fmicb.2019.01296
5. Tamburini G, Bommarco R, Cherico T, Kremen C, van der Heijden M, Liebman M, et al. Agricultural diversification promotes multiple ecosystem services without compromising yield. Sci Adv. (2020) 6:eaba1715. doi: 10.1126/sciadv.aba1715
6. Zhang K, Maltais-Landry G, Liao H. How soil biota regulate C cycling and soil C pools in diversified crop rotations. Soil Biol Biochem. (2021) 156:108219. doi: 10.1016/j.soilbio.2021.108219
7. Atreya K, Sharma R, Bajracharya N. Developing a sustainable agro-system for central Nepal using reduced tillage and straw mulching. J Environ Manag. (2008) 88:547–55. doi: 10.1016/j.jenvman.2007.03.017
8. Piotrowska-Długosz A, Lemanowicz J, Długosz J, Spychaj-Fabisiak E, Gozdowski D, Rybacki M. Spatio-temporal variations of soil properties in a plot scale: a case study of soil phosphorus forms and related enzymes. J Soils Sediments. (2016) 16:62–76. doi: 10.1007/s11368-015-1180-9
9. Barboza A, Pylro V, Jacques R, Gubiani P, de Quadros F, Trindade J, et al. Seasonal dynamics alter taxonomical and functional microbial profiles in Pampa biome soils under natural grasslands. PeerJ. (2018) 6:e4991. doi: 10.7717/peerj.4991
10. Shigyo N, Umeki K, Hirao T. Seasonal dynamics of soil fungal and bacterial communities in cool-temperate montane forests. Front Microbiol. (2019) 10:1944. doi: 10.3389/fmicb.2019.01944
11. Taye Z, Helgason B, Bell J, Norris C, Vail S, Robinson S, et al. Core and differentially abundant bacterial taxa in the rhizosphere of field grown brassica napus genotypes: implications for canola breeding. Front Microbiol. (2020) 10:3007. doi: 10.3389/fmicb.2019.03007
12. Fernandez-Gnecco G, Smalla K, Maccario L, Sørensen S, Barbieri P, Consolo V, et al. Microbial community analysis of soils under different soybean cropping regimes in the Argentinean south-eastern Humid Pampas. FEMS Microbiol Ecol. (2021) 97:fiab007. doi: 10.1093/femsec/fiab007
13. Fierer N. Embracing the unknown: Disentangling the complexities of the soil microbiome. Nat Rev Microbiol. (2017) 15:579–90. doi: 10.1038/nrmicro.2017.87
14. Compant S, Samad A, Faist H, Sessitsch A. A review on the plant microbiome: ecology, functions, and emerging trends in microbial application. J Adv Res. (2019) 19:29–37. doi: 10.1016/j.jare.2019.03.004
15. Hartmann M, Frey B, Mayer J, Mäder P, Widmer F. Distinct soil microbial diversity under long-term organic and conventional farming. ISME J. (2015) 9:1177–94. doi: 10.1038/ismej.2014.210
16. Benitez M, Osborne S, Lehman R. Previous crop and rotation history effects on maize seedling health and associated rhizosphere microbiome. Sci Rep. (2017) 7:15709. doi: 10.1038/s41598-017-15955-9
17. Babin D, Sommermann L, Chowdhury S, Behr J, Sandmann M, Neumann G, et al. Distinct rhizomicrobiota assemblages and plant performance in lettuce grown in soils with different agricultural management histories. FEMS Microbiol Ecol. (2021) 97:fiab027. doi: 10.1093/femsec/fiab027
18. Bziuk N, Maccario L, Douchkov D, Lueck S, Babin D, Sørensen S, et al. Tillage shapes the soil and rhizosphere microbiome of barley-but not its susceptibility towards Blumeria graminis f. sp. hordei. FEMS Microbiol Ecol. (2021) 97: fiab018. doi: 10.1093/femsec/fiab018
19. Degrune F, Theodorakopoulos N, Colinet G, Hiel M, Bodson B, Taminiau B, et al. Temporal dynamics of soil microbial communities below the seedbed under two contrasting tillage regimes. Front Microbiol. (2017) 8:1127. doi: 10.3389/fmicb.2017.01127
20. Sommermann L, Geistlinger J, Wibberg D, Deubel A, Zwanzig J, Babin D, et al. Fungal community profiles in agricultural soils of a long-term field trial under different tillage., fertilization and crop rotation conditions analyzed by high-throughput ITS-amplicon sequencing. PLoS ONE. (2018) 13:e0195345. doi: 10.1371/journal.pone.0195345
21. Babin D, Deubel A, Jacquiod S, Sørensen J, Geistlinger J, Grosch R, et al. Impact of long-term agricultural management practices on soil prokaryotic communities. Soil Biol Biochem. (2019) 129:17–28. doi: 10.1016/j.soilbio.2018.11.002
22. Jacquiod S, Franqueville L, Cécillon S, Vogel T, Simonet P. Soil bacterial community shifts after chitin enrichment: an integrative metagenomic approach. PLoS ONE. (2013) 8:e79699. doi: 10.1371/journal.pone.0079699
23. Verma J, Jaiswal D, Sagar R. Pesticide relevance and their microbial degradation: a-state-of-art. Rev Environ Sci Biotechnol. (2014) 13:429–66. doi: 10.1007/s11157-014-9341-7
24. Zhao Z, He J, Quan Z, Wu C, Sheng R, Zhang L, et al. Fertilization changes soil microbiome functioning, especially phagotrophic protists. Soil Biol Biochem. (2020) 148:107863. doi: 10.1016/j.soilbio.2020.107863
25. Villamil MB, Kim N, Riggins CW, Zabaloy MC, Allegrini M, Rodríguez-Zas SL. Microbial signatures in fertile soils under long-term n management. Front Soil Sci. (2021) 1:765901. doi: 10.3389/fsoil.2021.765901
26. van der Putten W, Bardgett R, Bever J, Bezemer T, Casper B, Fukami T, et al. Plant-soil feedbacks: the past, the present and future challenges. J Ecol. (2013) 101:265–76. doi: 10.1111/1365-2745.12054
27. Lapsansky E, Milroy A, Andales M, Vivanco J. Soil memory as a potential mechanism for encouraging sustainable plant health and productivity. Curr Opin Biotech. (2016) 38:137–42. doi: 10.1016/j.copbio.2016.01.014
28. Deubel A, Hofmann B, Orzessek D. Long-term effects of tillage on stratification and plant availability of phosphate and potassium in a loess chernozem. Soil Tillage Res. (2011) 117:85–92. doi: 10.1016/j.still.2011.09.001
29. Meier U,. Growth Stages of Mono- Dicotyledonous Plants: BBCH Monograph: Open Agrar Repositorium. (2001). Available online at: https://www.politicheagricole.it/flex/AppData/WebLive/Agrometeo/MIEPFY800/BBCHengl2001.pdf (accessed October 10, 2021).
31. Suzuki M, Taylor L, De Long E. Quantitative analysis of small-subunit rRNA genes in mixed microbial populations via 5'-nuclease assays. Appl Environ Microbiol. (2000) 4605–14. doi: 10.1128/AEM.66.11.4605-4614.2000
32. Vogel C, Babin D, Pronk G, Heister K, Smalla K, Kögel-Knabner I. Establishment of macro-aggregates and organic matter turnover by microbial communities in long-term incubated artificial soils. Soil Biol Biochem. (2014) 79:57–67. doi: 10.1016/j.soilbio.2014.07.012
33. Gschwendtner S, Reichmann M, Müller M, Radl V, Munch JC, Schloter M. Effects of genetically modified amylopectin-accumulating potato plants on the abundance of beneficial and pathogenic microorganisms in the rhizosphere. Plant Soil. (2010) 335:413–22. doi: 10.1007/s11104-010-0430-2
34. White T, Bruns T, Lee S, Taylor J. Amplification and direct sequencing of fungal ribosomal RNA genes for phylogenetics. In: M Innis, D Gelfand, J Sninsky, T White, editors, PCR Protocols: A Guide to Methods and Applications. New York, NY: Academic Press (1990). p. 315–22. doi: 10.1016/B978-0-12-372180-8.50042-1
35. Yu Y, Lee C, Kim J, Hwang S. Group-specific primer and probe sets to detect methanogenic communities using quantitative real-time polymerase chain reaction. Biotechnol Bioeng. (2005) 89:670–9. doi: 10.1002/bit.20347
36. Sundberg C, Al-Soud W, Larsson M, Alm E, Yekta S, Svensson B, et al. 454 pyrosequencing analyses of bacterial and archaeal richness in 21 full-scale biogas digesters. FEMS Microbiol Ecol. (2013) 85:612–26. doi: 10.1111/1574-6941.12148
37. Caporaso J, Lauber C, Walters W, Berg-Lyons D, Lozupone C, Turnbaugh P, et al. Global patterns of 16S rRNA diversity at a depth of millions of sequences per sample. Proc Natl Acad Sci USA. (2011) 108(Suppl.1):4516-22. doi: 10.1073/pnas.1000080107
38. Ihrmark K, Bödeker I, Cruz-Martinez K, Friberg H, Kubartova A, Schenck J, et al. New primers to amplify the fungal ITS2 region – evaluation by 454-sequencing of artificial and natural communities. FEMS Microbiol Ecol. (2012) 82:666–77. doi: 10.1111/j.1574-6941.2012.01437.x
39. Martin M. Cutadapt removes adapter sequences from high-throughput sequencing reads. EMBnet J. (2011) 17:10–2. doi: 10.14806/ej.17.1.200
40. Callahan B, McMurdie P, Rosen M, Han A, Johnson A, Holmes S. DADA2: high-resolution sample inference from Illumina amplicon data. Nat Methods. (2016) 13:581–3. doi: 10.1038/nmeth.3869
41. Bolyen E, Rideout J, Dillon M, Bokulich N, Abnet C, Al-Ghalith G, et al. Reproducible, interactive, scalable and extensible microbiome data science using QIIME 2. Nat Biotechnol. (2019) 37:852–7. doi: 10.1038/s41587-019-0209-9
42. Quast C, Pruesse E, Yilmaz P, Gerken J, Schweer T, Yarza P, et al. The SILVA ribosomal RNA gene database project: improved data processing and web-based tools. Nucleic Acids Res. (2013) 41:D590–6. doi: 10.1093/nar/gks1219
43. Nilsson R, Larsson K, Taylor A, Bengtsson-Palme J, Jeppesen T, Schigel D, et al. The UNITE database for molecular identification of fungi: handling dark taxa and parallel taxonomic classifications. Nucleic Acids Res. (2019) 47:D259–64. doi: 10.1093/nar/gky1022
44. Davis N, Proctor D, Holmes S, Relman D, Callahan B. Simple statistical identification and removal of contaminant sequences in markergene and metagenomics data. Microbiome. (2018) 6:226. doi: 10.1186/s40168-018-0605-2
45. Anderson M. A new method for non-parametric multivariate analysis of variance. Austral Ecol. (2001) 26:32–46. doi: 10.1046/j.1442-9993.2001.01070.x
46. Nguyen NH, Song Z, Bates ST, Branco S, Tedersoo L, Menke J, et al. FUNGuild: an open annotation tool for parsing fungal community datasets by ecological guild. Fungal Ecol. (2016) 20:241–8. doi: 10.1016/j.funeco.2015.06.006
47. Oksanen J, Blanchet F, Friendly M. Vegan: Community Ecology Package. R package version 2.5-6. (2019). Available online at: https://cran.r-project.org/web/packages/vegan/index.html (accessed January 18, 2021).
48. De Mendiburu F. Agricolae: Statistical Procedures for Agricultural Research. R package version 1.3-2. (2020). Available online at: https://CRAN.R-project.org/package=agricolae (accessed March 26, 2021).
49. Mangiafico S. Rcompanion: Functions to Support Extension Education Program Evaluation. R package version 2.3.26. (2020). Available online at: https://CRAN.R-project.org/package=rcompanion (accessed February 26, 2021).
51. Robinson M, McCarthy D, Smyth G. edgeR: a bioconductor package for differential expression analysis of digital gene expression data. Bioinformatics. (2010) 26:139–40. doi: 10.1093/bioinformatics/btp616
52. Wickham H. ggplot2: Elegant Graphics for Data Analysis. New York, NY: Springer-Verlag New York. (2016). Available online at: https://ggplot2.tidyverse.org (accessed February 24, 2021).
53. Juggins S,. Rioja: Analysis of Quaternary Science Data, R package version (0.9-21). (2017). Available online at: http://cran.r-project.org/package=rioja (accessed February 18, 2021).
54. McMurdie P, Holmes S. phyloseq: an R package for reproducible interactive analysis and graphics of microbiome census data. PLoS ONE. (2013) 8:e61217. doi: 10.1371/journal.pone.0061217
55. Roberts D. labdsv: Ordination Multivariate Analysis for Ecology. R package version 2.0-1. (2019). Available online at: https://CRAN.R-project.org/package=labdsv (accessed March 10, 2021).
56. Wang Y, Naumann U, Eddelbuettel D, Wilshire J, Warton D, Byrnes J, et al. mvabund: Statistical Methods for Analysing Multivariate Abundance Data. R package version 4.1.3. (2020). Available online at: https://CRAN.R-project.org/package=mvabund (accessed March 12, 2021).
57. Kolde R. pheatmap: Pretty Heatmaps. R package version 1.0.12.2019. (2019). Available online at: https://CRAN.R-project.org/package=pheatmap (accessed March 26, 2021).
58. Morgan M,. BiocManager: Access the Bioconductor Project Package Repository. R package version 1.30.10. (2019). Available online at: https://CRAN.R-project.org/package=BiocManager (accessed January 18, 2021).
59. Chen H, Boutros P. VennDiagram: a package for the generation of highly-customizable Venn and Euler diagrams in R. BMC Bioinformatics. (2011) 12:35. doi: 10.1186/1471-2105-12-35
60. Carbonetto B, Rascovan N, Álvarez R, Mentaberry A, Vázquez M. Structure, composition and metagenomic profile of soil microbiomes associated to agricultural land use and tillage systems in Argentine Pampas. PLoS ONE. (2014) 9:e99949. doi: 10.1371/journal.pone.0099949
61. Srour A, Ammar H, Subedi A, Pimentel M, Cook R, Bond J, et al. Microbial communities associated with long-term tillage and fertility treatments in a corn-soybean cropping system. Front Microbiol. (2020) 11:1363. doi: 10.3389/fmicb.2020.01363
62. Six J, Elliott E, Paustian K. Soil macroaggregate turnover and microaggregate formation: a mechanism for C sequestration under no-tillage agriculture. Soil Biol Biochem. (2000) 32:2099–103. doi: 10.1016/S0038-0717(00)00179-6
63. Or D, Keller T, Schlesinger W. Natural and managed soil structure: on the fragile scaffolding for soil functioning. Soil Tillage Res. (2021) 208:104912. doi: 10.1016/j.still.2020.104912
64. Schlüter S, Großmann C, Diel J, Wu G, Tischer S, Deubel A, et al. Long-term effects of conventional and reduced tillage on soil structure, soil ecological and soil hydraulic properties. Geoderma. (2018) 332:10–9. doi: 10.1016/j.geoderma.2018.07.001
66. Geisseler D, Scow K. Long-term effects of mineral fertilizers on soil microorganisms – a review. Soil Biol Biochem. (2014) 75:54–63. doi: 10.1016/j.soilbio.2014.03.023
67. Shanmugam G, Buehring N, Prevost J, Kingery W. Soil bacterial community diversity and composition as affected by tillage intensity treatments in corn-soybean production systems. Microbiol Res. (2021) 12:157–72. doi: 10.3390/microbiolres12010012
68. Lennartsson P, Taherzadeh M, Edebo L. Rhizopus. In: C Batt, M Tortorello, editors, Encyclopedia of Food Microbiology. 2nd ed. New York, NY: Academic Press (2014). p. 284–90. doi: 10.1016/B978-0-12-384730-0.00391-8
69. Yadav D, Mir N, Wadhwa R, Tushir S, Sethi S, Anurag R, et al. Hydrolysis of peanut (Arachis hypogea L.) protein concentrate by fungal crude protease extract: effect on structural., functional and in-vitro protein digestibility. J Food Sci Technol. (2021) 21:5225. doi: 10.1007/s13197-021-05225-y
70. Fierer N, Bradford M, Jackson R. Toward an ecological classification of soil bacteria. Ecology. (2007) 88:1354–64. doi: 10.1890/05-1839
71. Barelli L, Waller A, Behie S, Bidochka M. Plant microbiome analysis after Metarhizium amendment reveals increases in abundance of plant growth-promoting organisms and maintenance of disease-suppressive soil. PLoS ONE. (2020) 15:e0231150. doi: 10.1371/journal.pone.0231150
72. Patel T. Metarhizium. In: N Amaresan, M Senthil Kumar, K Annapurna, K Kumar, A Sankaranarayanan, editors, Beneficial Microbes in Agro-Ecology. New York, NY: Academic Press (2020). p. 593–610. doi: 10.1016/B978-0-12-823414-3.00029-0
73. Gosling P, Hodge A, Goodlass G, Bending G. Arbuscular mycorrhizal fungi and organic farming. Agric Ecosyst Env. (2006) 113:17–35. doi: 10.1016/j.agee.2005.09.009
74. Covacevich F, Echeverría H, Aguirrezábal L. Soil available phosphorus status determines indigenous mycorrhizal colonization of field and glasshouse-grown spring wheat from Argentina. Appl Soil Ecol. (2007) 35:1–9. doi: 10.1016/j.apsoil.2006.06.001
75. Schalamuk S, Cabello M. Arbuscular mycorrhizal fungal propagules from tillage and no-tillage systems: possible effects on Glomeromycota diversity. Mycologia. (2010) 102:261–8. doi: 10.3852/08-118
76. Pereyra S, Dill-Macky R, Sims A. Survival and inoculum production of Gibberella zeae in wheat residue. Plant Dis. (2004) 88:724–30. doi: 10.1094/PDIS.2004.88.7.724
77. Wahdan S, Hossen S, Tanunchai B, Schädler M, Buscot F, Purahong W. Future climate significantly alters fungal plant pathogen dynamics during the early phase of wheat litter decomposition. Microorganisms. (2020) 8:908. doi: 10.3390/microorganisms8060908
78. Lidbury I, Borsetto C, Murphy A, Bottrill A, Jones A, Bending G, et al. Niche-adaptation in plant-associated Bacteroidetes favours specialisation in organic phosphorus mineralisation. ISME J. (2021) 15:1040–55. doi: 10.1038/s41396-020-00829-2
79. Wieczorek AS, Schmidt O, Chatzinotas A, von Bergen M, Gorissen A, Kolb S. Ecological functions of agricultural soil bacteria and microeukaryotes in chitin degradation: a case study. Front Microbiol. (2019) 10:1293. doi: 10.3389/fmicb.2019.01293
80. Zare R, Gams W, Starink-Willemse M, Summerbell R. Gibellulopsis, a suitable genus for Verticillium nigrescens, and Musicillium, a new genus for V. theobromae. Nova Hedwigia. (2007) 85:463–89. doi: 10.1127/0029-5035/2007/0085-0463
81. Klosterman S, Subbarao K, Kang S, Veronese P, Gold S, Thomma B, et al. Comparative genomics yields insights into niche adaptation of plant vascular wilt pathogens. PLoS Patholog. (2011) 7:e1002137. doi: 10.1371/journal.ppat.1002137
82. Beale R, Pitt D. The antifungal properties of Minimedusa polyspora. Mycol Res. (1995) 99:337–42. doi: 10.1016/S0953-7562(09)80910-6
Keywords: tillage practice, fertilization intensity, high-throughput amplicon sequencing, 16S rRNA gene, fungal ITS2 region
Citation: Fernandez-Gnecco G, Covacevich F, Consolo VF, Behr JH, Sommermann L, Moradtalab N, Maccario L, Sørensen SJ, Deubel A, Schellenberg I, Geistlinger J, Neumann G, Grosch R, Smalla K and Babin D (2022) Effect of Long-Term Agricultural Management on the Soil Microbiota Influenced by the Time of Soil Sampling. Front. Soil Sci. 2:837508. doi: 10.3389/fsoil.2022.837508
Received: 16 December 2021; Accepted: 07 February 2022;
Published: 31 March 2022.
Edited by:
Patricia Dorr De Quadros, University of Waterloo, CanadaReviewed by:
Simone Raposo Cotta, University of São Paulo, BrazilDaniel Kumazawa Morais, Academy of Sciences of the Czech Republic (ASCR), Czechia
Copyright © 2022 Fernandez-Gnecco, Covacevich, Consolo, Behr, Sommermann, Moradtalab, Maccario, Sørensen, Deubel, Schellenberg, Geistlinger, Neumann, Grosch, Smalla and Babin. This is an open-access article distributed under the terms of the Creative Commons Attribution License (CC BY). The use, distribution or reproduction in other forums is permitted, provided the original author(s) and the copyright owner(s) are credited and that the original publication in this journal is cited, in accordance with accepted academic practice. No use, distribution or reproduction is permitted which does not comply with these terms.
*Correspondence: Doreen Babin, doreen.babin@julius-kuehn.de